It is a capital mistake to theorize before one has data
- Sherlock Holmes in ‘A Study in Scarlet’ by Arthur Conan Doyle
When one of the most famous fictional detectives in history who is known for his analysis & deduction prowess makes this statement, it reaffirms the power of data. What Mr. Holmes is trying to tell us is that theories in the absence of data and its analysis are useless. And now that we have the famed Sherlock Holmes’ approval, let’s embark on ‘A Study in Data Processing’ in this blog.
The value of data in today’s world
Data is nothing but pieces of information - both quantitative and qualitative. Data has been critical for humankind right from the ancient ages - as early as the Paleolithic age when bones were used to record data.
As humankind evolved, so did the methods of collecting & recording data. The advent of the Internet followed by the proliferation of personal, portable devices accelerated the rate at which data was produced, collected & analyzed. Data has now become an invaluable asset for businesses, enabling them to make decisions based on facts and to focus on what matters the most. In today’s day and age, businesses irrespective of verticals or size, cannot afford to ignore data.
Flooded by data but starved for insights. Why data processing is critical
Mathematician and best-selling author John Allen Paulo has famously and aptly said:
‘Data, data everywhere, but not a thought to think
There is a profusion of data everywhere, making it even more critical to analyze it and make sense of it. A study by specialists has concluded that businesses are losing $5.2 million in revenue due to untapped data. It becomes obvious then that data collection is of no use unless that data is processed, analyzed and acted upon.
So what is data processing? Data processing in simple terms is the turning of raw data into usable information that can be acted upon. There are six stages in the Data Processing Cycle where raw data (input) is fed into a system to produce actionable insights (output). Each step is taken in a specific order, but the entire process is repeated in a cyclic manner until the desired output is achieved.
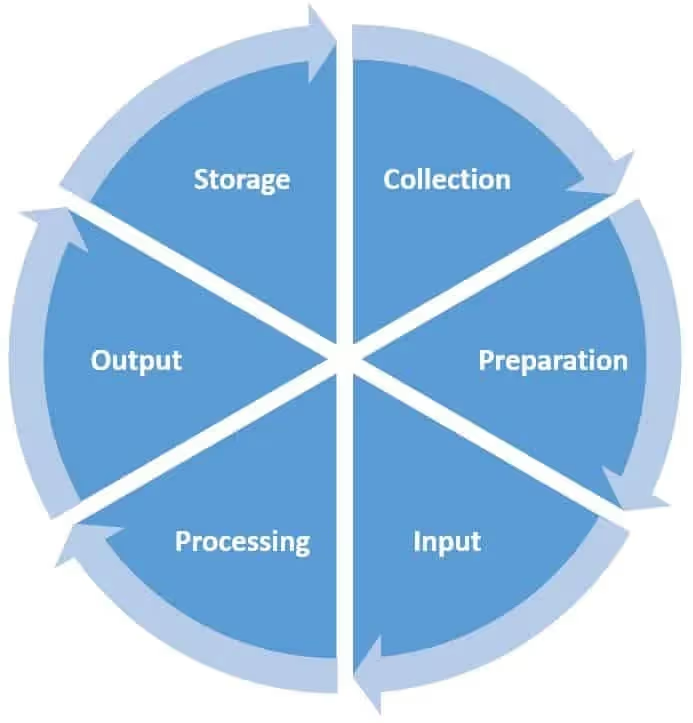
Now that we have seen how Data Processing is broken down into stages, let’s look at the different ways data processing is done.
To batch process, or to stream process? - That is the question
In today’s era of Big Data, there are two primary ways of data processing - Batch Processing and Stream Processing. There is an ongoing debate on which of the two ways of processing large volumes of data is better. Both approaches have their pros & cons, and eventually the choice for your pick should be based on your use case. Let’s look a little deeper into both and understand some real world use cases best suited for both respectively.
Batch processing
The batch processing model, as the name suggests, takes a set of data collected over time (a batch) and sends it for processing. Batch processing is often used when dealing with large volumes of data or data sources from legacy systems. Batch data may also require all the data needed for the batch to be loaded to some type of storage, a database or file system to then be processed. Batch processing does not immediately feed data into an analytics system, so results are not available in real-time. Typically, batch processing jobs are run at regularly scheduled times e.g. at the end of a day or at the end of a month.
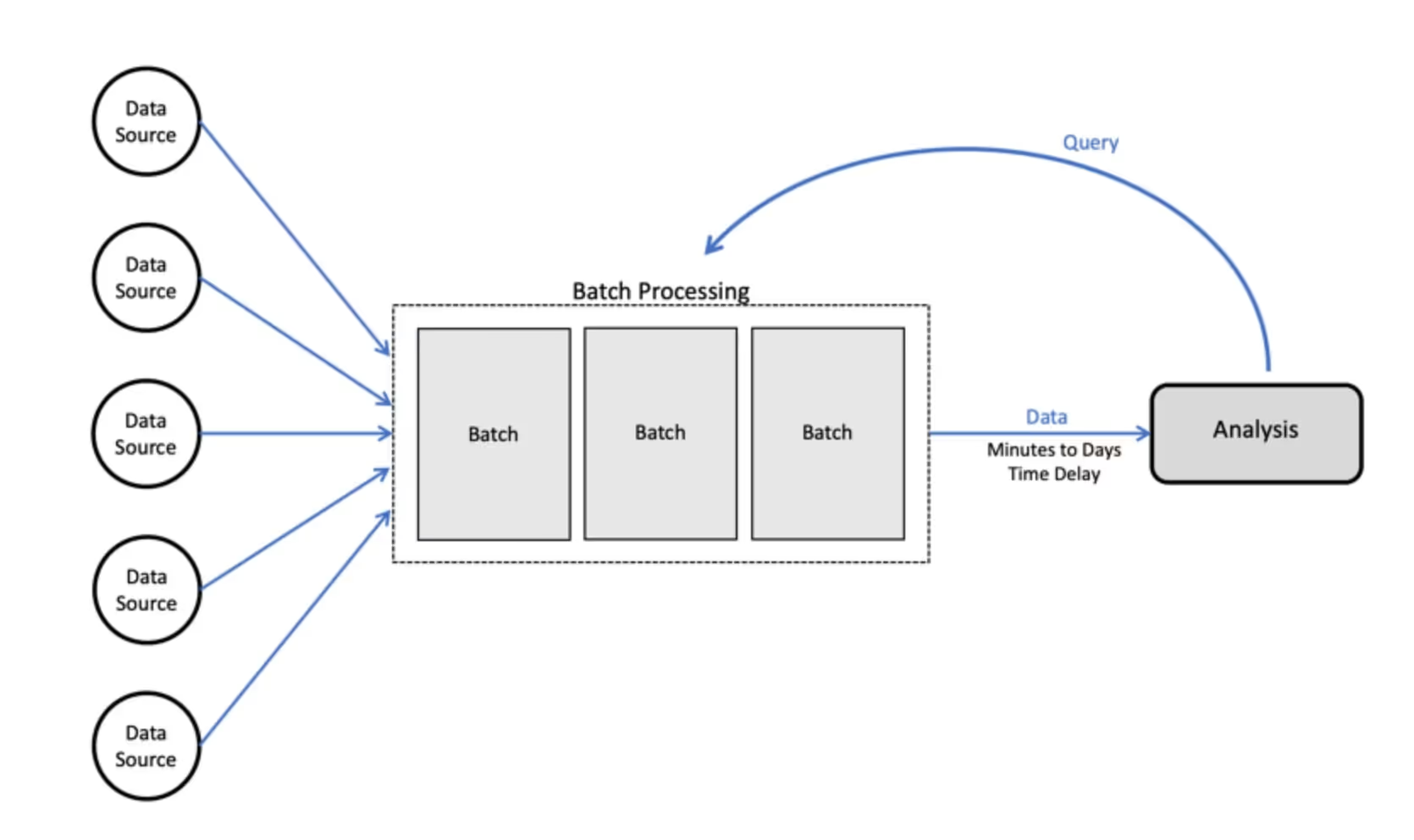
To summarize, you should consider batch processing when:
- Real-time insights are not a requirement
- You get access to the data in batches rather than in streams
- You are working with large datasets and are running a complex algorithm
- The algorithm requires access to the entire batch
For example, if you’re trying to correlate the effect of your customers upgrading their plan to increased usage of certain features, you might want to join a couple of tables & run some analytics queries. If this is done at the end of the day rather than as soon as customers upgrade, you may have a daily report or graphs that may suffice for your requirement. In this case, batch processing would work for you.
Stream Processing
Stream Processing deals with continuously ingested data and processing data as soon as it arrives in the storage layer. This would often also be very close to the time the data was generated. Because stream processing is in charge of processing data in motion and providing analytics results quickly, it generates near-instant results. For the end user the processing happens in real-time, thus turning big data into fast data.

To summarize, you should consider stream processing when:
- Your data sources are generating data continuously and at high speed
- Your use case demands action be taken on the data near real-time, as best as it can
For example, if you want to analyze your stream of events for fraud prevention, you may need to continuously process the events to detect any fraudulent activity and alert or stop the transaction in near real time. Another example could be of a company that constantly tracks its social media feeds to gauge the public sentiment about their brand and respond to it quickly. This makes the company much more agile and provides a competitive edge.
Stream processing and real-time processing may seem synonymous, yet they have subtle and important differences.
Micro-batch Processing.
Somewhere between the domains of Batch and Stream Processing lies Micro-batch Processing.
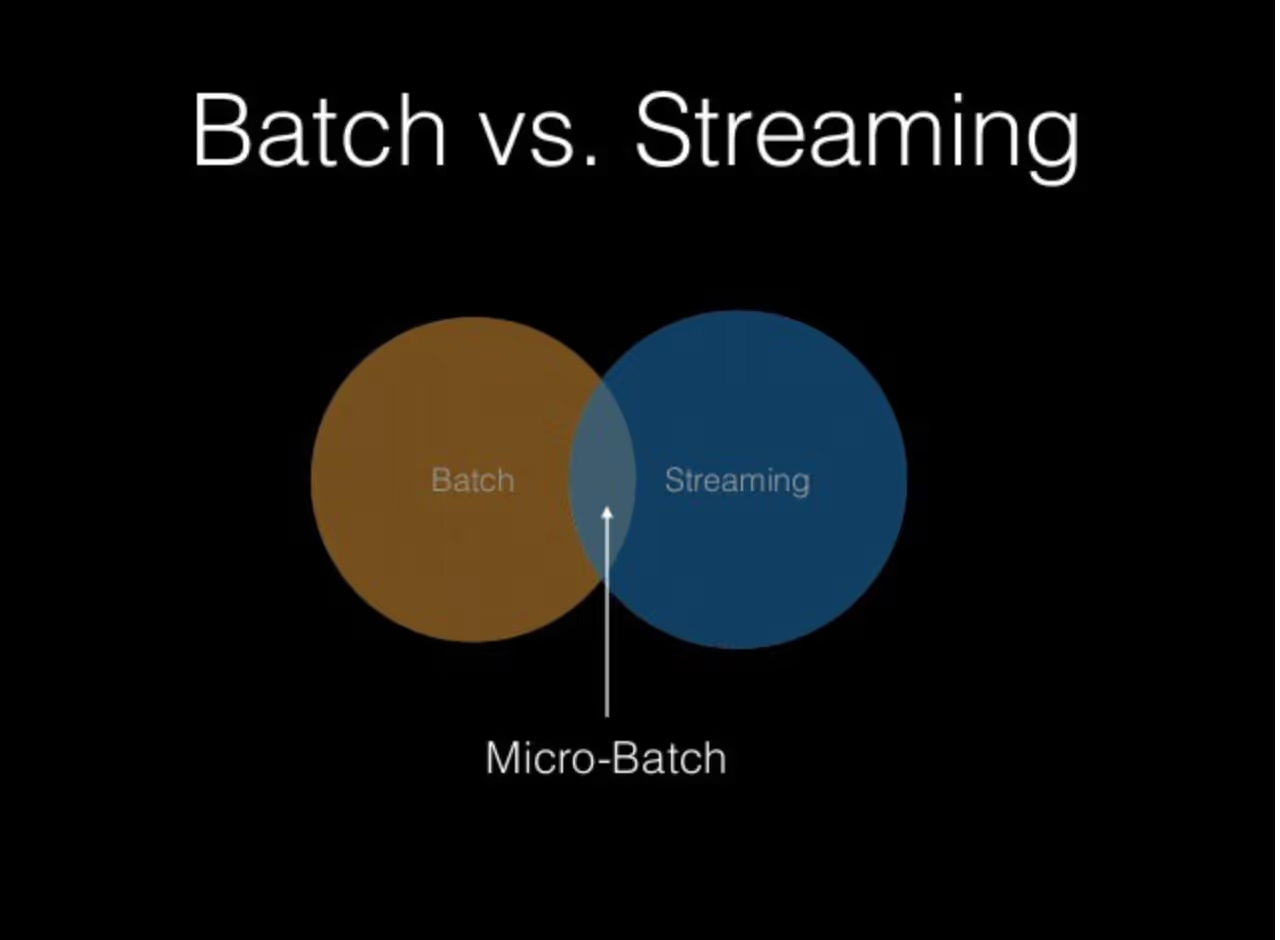
This is a special case of batch processing where the processing is run on much smaller batches of data - typically less than a minute’s worth of data. In practice, there is little difference between stream processing and micro-batch processing as data is picked in windows of batches while processing the stream of events.
Micro-batch processing is useful when we need very fresh data, but not necessarily real-time. An hour or a day may be too much to wait for in this case, but a few seconds of delay is acceptable.
For example, user behavior data that is continuously flowing from an e-commerce website would be a good use case for micro-batch. Any changes to the website which have resulted in changed user behavior might be in need for attention - a day’s time might be too long, but a minute’s wait is acceptable.
Conclusion
So is there a winner between these two types of data processing then?
It’s evident that what you choose depends entirely on your use case. You may even opt to go for a combination of the two. The winner is none other than the smart team that understands their use case and picks what suits them best at that point in time.
The conclusion then is just what our friend Sherlock Holmes was fond of saying often - “Elementary, my dear Watson”.
Explore More Blogs
If you enjoyed this blog, here are some other topics you might find interesting:
- Understanding Continuous Integration and Continuous Delivery (CI/CD)
- The challenges of being the only finance person in a team of Techies
- Best way to grow your SaaS business using cold emails
- Deno vs Node - a side by side comparison
- Self Managed Vs Managed Kafka Service
- Why Load Test Often & Early: Our Experiments with Gatling
- Deep Dive Into ClickHouse
- What is HAR File?
- Benefits of Dogfooding with Zipy